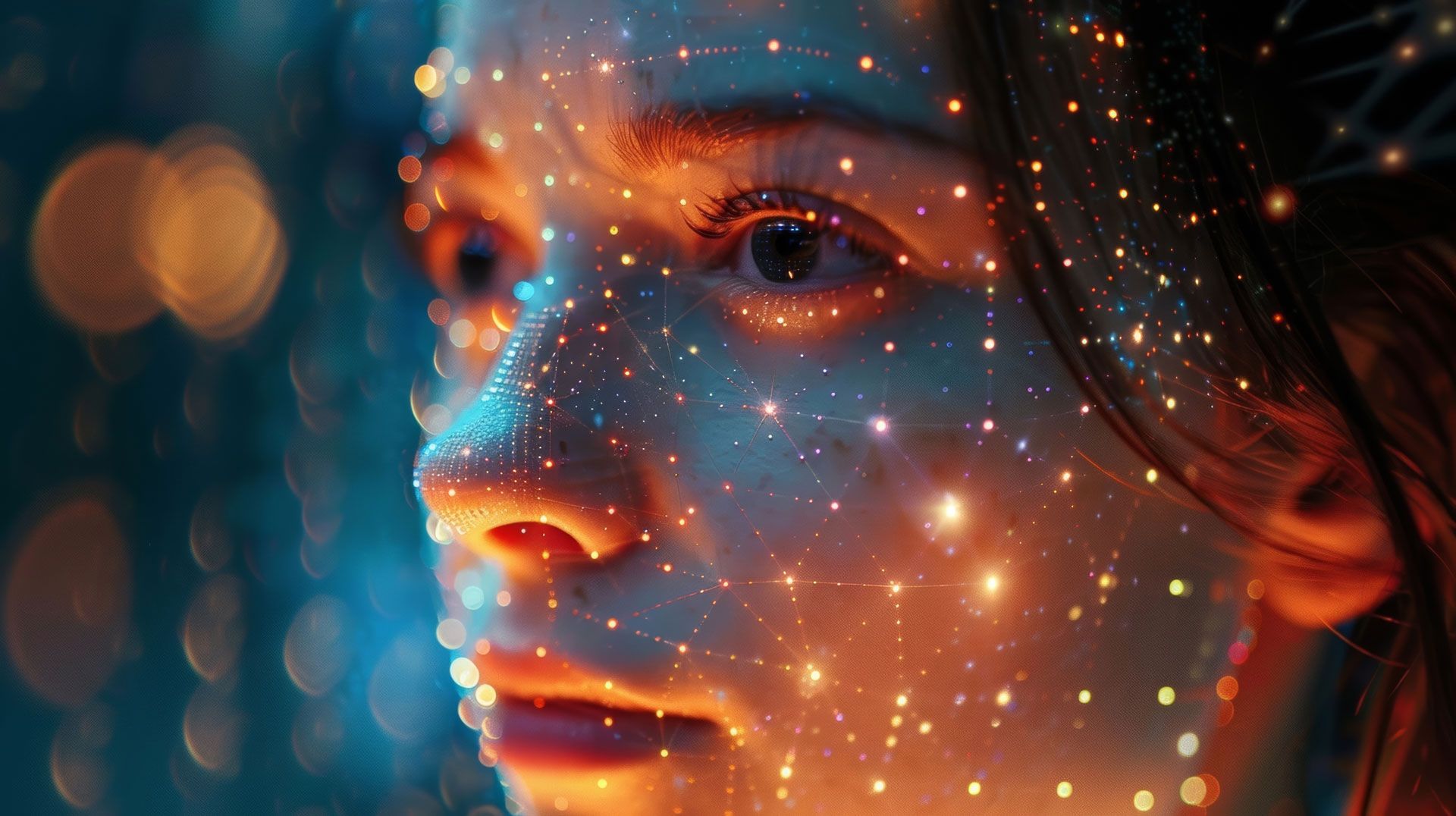
Detecting deepfakes: Generative AI uptake casts doubt on multimedia content
By Alessandra Sala, Senior Director of AI and Data Science, Shutterstock
The explosive rise of artificial intelligence (AI) technologies with deep learning capabilities has escalated risks in the digital world to unprecedented levels.
Synthetic AI-generated media – known for short as “deepfakes” – are spreading misinformation and becoming increasingly hard to detect, either by the human eye or by existing cybersecurity systems.
Deepfakes consisting of video, images, text, and audio materials have blurred the lines between what is real and fake.
In response, governments and international organizations are striving to set out practical policy measures, codes of conduct and regulations to enhance the security and trust of AI systems.
Building trust in digital systems
Deepfakes can be used for harmless purposes such as social media memes. Increasingly, however, cybercriminals can exploit or imitate voices and video images to breach security barriers.
One way is impersonating executives or staff in video or audio format and effectively stealing their credentials. Such attacks can target automated systems that authenticate based on voice recognition, as well as employees themselves.
Political leaders could also potentially be targeted this way, with far-reaching international consequences.
While deepfakes are a threat in both developed and developing countries, the danger can be exacerbated in countries with low levels of digital literacy. Manipulated content can, for example, reinforce societal biases and stereotypes, trigger gender-based violence, or escalate ethnic, religious, and political divisions.
Safeguarding authenticity in the age of generative AI
With the growing prevalence of generative AI, human content creators may struggle to attest and defend ownership of their works.
Verifying the authenticity and ownership of multimedia assets is vital to protect the digital rights of people and companies alike.
The provenance of data used to train AI models is equally crucial from the perspective of maintaining transparency. Knowing where data comes from helps to establish the authenticity and reliability of AI-generated content – and anticipate potential issues surrounding accuracy, bias, or licensing.
Generative AI developers are creating training datasets using material that has been scraped from the web, often without clarity about the consent of copyright holders. The trained AI model will subsequently generate new content based on those materials, potentially infringing on the rights of the copyright holder.
For now, not much can be done to protect copyrighted works from being fed into generative AI models under training.
Still, developers should ensure that they remain in compliance with applicable laws on data acquisition. The European Union’s new AI act, for instance, requires AI systems to be transparent and comply with copyright legislation.
Developers seeking to enrich their AI training data, therefore, may need to compensate intellectual property owners, either through licensing or revenue sharing.
Consumers, in turn, should be able to determine whether AI systems were trained on protected content, review terms of service and privacy policies, and avoid generative AI tools that lack official licensing or clear compliance with open-source licenses.
Opt-ins and -outs
Companies mindful of intellectual property concerns are giving artists the opportunity to optout of their work being used to train AI image generators, with opt-out decisions being reflected by the next iteration of the image generator.
But this still leaves the onus on content creators to protect their intellectual property, rather than requiring AI developers to secure intellectual property rights before using any pre-existing work.
Instead, companies should require the creator’s opt-in from the very beginning.
Going forward, ethical AI developers would provide mechanisms to disclose the provenance of AI-generated content, with full transparency about all content that went into the training data.
Such information would make authenticity verifiable. It would also protect business users of AI-generated content from the risk of intellectual property infringement.
Multimedia authenticity protocols and watermarking call for widely recognized international technical standards. This is a field with significant opportunities for collaboration among AI developers and regulators, as well as academia, businesses, content creators, and AI users of all kinds.
During the AI for Good Global Summit, a full-day workshop dives into multimedia authenticity issues, with a focus on international standards, the use of watermarking technology, enhanced security protocols, and cybersecurity awareness.
The free workshop, open to anyone interested, takes place on 31 May in Geneva, Switzerland, and online.
The session aims to unite leading minds and experts to discuss recent research findings and ongoing standardization efforts, aiming to create a collaborative platform to collectively address current gaps. It also aims to develop recommendations for practical actions and encourage further investment in this field.
Register for the workshop here: Detecting deepfakes and Generative AI: Standards for AI watermarking and multimedia authenticity.
Learn more about the AI for Good Global Summit.
In another blog post, Alessandra Sala delves into digital watermarks and how they can ensure authenticity in AI-generated multimedia content.
Header image credit: Adobe Stock/AI generated