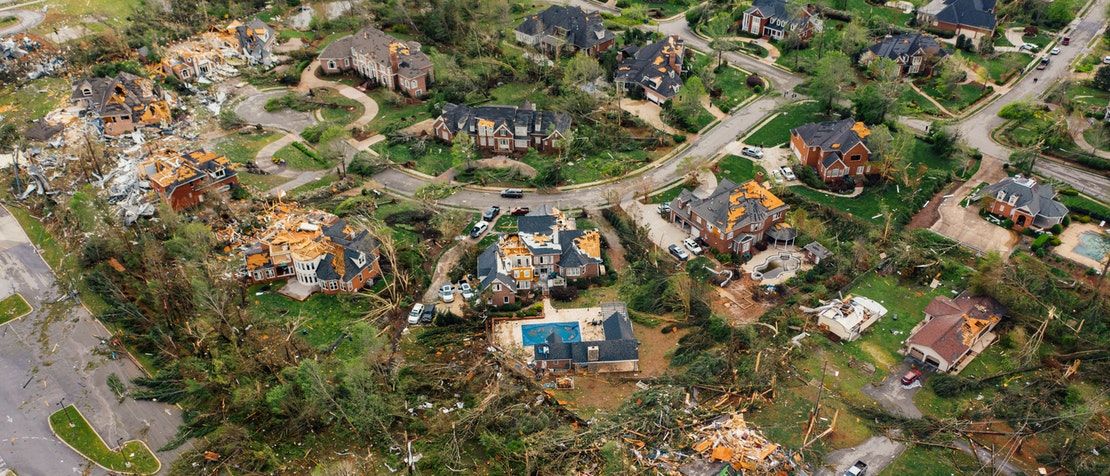
Here’s why effective disaster management needs responsible AI
The use of artificial intelligence holds promise in helping avert, mitigate and manage disasters by analyzing swaths of data, but more efforts are required to ensure that technologies are deployed in a responsible, equitable manner. According to UNDDR, about 1.2 million lives have been lost worldwide and more than 4 billion people affected in disasters that took place between 2000 and 2019. During a webinar held as part of the year-round AI for Good Global Summit, experts in technology and humanitarian action examined the most pressing questions emerging from the use of AI solutions to prepare for and respond to disasters.
Faster data labelling
Cameron Birge, Senior Program Manager Humanitarian Partnerships at Microsoft, says their work in using AI for humanitarian missions has been human-centric. “Our approach has been about helping the humans, the humans stay in the loop, do their jobs better, faster and more efficiently,” he noted. One of their projects in India uses roofing as a proxy indicator of households with lower incomes who are likely to be more vulnerable to extreme events like typhoons. Satellite imagery analysis of roofs are used to inform disaster response and resilience-building plans. A simple yet rewarding avenue of using AI has been around data labelling to train AI models to assist disaster management.
“Instead of replacing all the manual labelling that takes place, AI helps speed it up,” said Birge.
One challenge, he noted, has been around “unbiased, good, clean, trusted data”. He also encouraged humanitarian organizations to understand their responsibilities when making use of AI models to support decision-making. “You have to ensure you sustain, train and monitor these models,” he advised. Microsoft also wants to promote more sharing of data with its ‘Open Data’ campaign.
Precise decision support
AI is becoming increasingly important to the work of the World Meteorological Organization (WMO). Supercomputers crunch petabytes of data to forecast weather around the world. The WMO also coordinates a global programme of surface-based and satellite observations. Their models merge data from more than 30 satellite sensors, weather stations and ocean-observing platforms all over the planet, explained Anthony Rea, Director of the Infrastructure Department at WMO. AI can help interpret resulting data and help with decision support for forecasters who receive an overwhelming amount of data, said Rea. “We can use AI to recognize where there might be a severe event or a risk of it happening, and use that in a decision support mechanism to make the forecaster more efficient and maybe allow them to pick up things that couldn’t otherwise be picked up.” Understanding the potential impact of extreme weather events on an individual or a community and assessing their vulnerability requires extra information on the built environment, population, and health.
“To enable AI to create an environment where it can thrive, data needs to be open, available and interoperable,” Rea pointed out.
“We need to understand where AI and machine learning can help and where we are better off taking the approach of a physical model. There are many examples of that case as well. Data curation is really important,” he added. WMO also sets the standards for international weather data exchange, including factors such as identifying the data, formats, and ontologies. While advocating for the availability of data, Rea also highlighted the need to be mindful of privacy and ethical considerations when dealing with personal data. WMO is revising its own data policies ahead of its Congress later this year, committing to free and open exchange of data beyond the meteorological community.
‘Not a magic bullet’
Rea believes that AI cannot replace the models built on physical understanding and decades of research into interactions between the atmosphere and oceans. “One of the things we need to guard against in the use of AI is to think of it as a magic bullet,” he cautioned. Instead of vertically integrating a specific dataset and using AI to generate forecasts, Rea sees a lot of promise in bringing together different datasets in a physical model to generate forecast information. “We use machine learning and AI in situations where maybe we don’t understand the underlying relationships. There are plenty of places in our area of science and service delivery where that is possible.” Rakesh Bharania, Director of Humanitarian Impact Data at Salesforce.org, also sees the potential of artificial or augmented intelligence in decision support and areas where a lot of contextual knowledge is not required. “If you have a lot of data about a particular problem, then AI is certainly arguably much better than having humans going through that same mountain of data. AI can do very well in answering questions where there is a clear, right answer,” he said. One challenge in the humanitarian field, Bharania noted, is scaling a solution from a proof of concept to something mature, usable, and relevant. He also cautioned that data used for prediction is not objective and can impact results.
Bharania urged humanitarian organizations keen to explore innovative technologies to own the hard questions about ‘go and no-go’ areas instead of leaving them solely to technology partners.
“It’s going to be a collaboration between the private sector who typically are the technology experts and the humanitarians who have the mission to come together and actually focus on determining what the right applications are, and to do so in an ethical and effective and impactful manner,” he said. Networks such as NetHope and Impactcloud are trying to build that space of cross-sectoral collaboration, he added.
Towards ‘white box AI’
Yasunori Mochizuki, NEC Fellow at NEC Corporation, recalled how local governments in Japan relied on social networks and crowd-behaviour analyses for real-time decision-making in the aftermath of 2011’s Great East Japan Earthquake and resulting tsunami. Their solution analyzed tweets to extract information and identify areas with heavy damage and need for immediate rescue, and integrated it with information provided by public agencies. “Tweets are challenging for computers to understand as the context is heavily compressed and expression varies from one user to another. It is for this reason that the most advanced class of natural language processing AI in the disaster domain was developed,” Mochizuki explained. Mochizuki sees the need for AI solutions in disaster risk reduction to provide management-oriented support, such as optimizing logistics and recovery tasks. This requires “white box AI” he said, also known as ‘explainable AI’. “While typical deep learning technology doesn’t tell us why a certain result was obtained, white box AI gives not only the prediction and recommendation, but also the set of quantitative reasons why AI reached the given conclusion,” he said.
The explainable AI approach could enable transparent and responsible use of technologies for more inclusive, comprehensive disaster risk management, Mochizuki added.
Webinar host and moderator Muralee Thummarukudy, Operations Manager, Crisis Management Branch at the United Nations Environment Programme (UNEP), also acknowledged the value of explainable AI. “It will be increasingly important that AI is able to explain the decisions transparently so that those who use or are subject to the outcome of these black box technologies would know why those decisions were taken,” he said.
Join ITU’s new Focus Group
The webinar followed an earlier session on the use of AI to reduce disaster risks, delving further into a holistic strategy for risk reduction, preparedness, response and recovery. “Inefficiencies in data collection and processing can have serious consequences adversely impacting the speed and effectiveness of interventions to save lives,” said Bilel Jamoussi, Chief of the Study Groups Department, Telecommunication Standardization Bureau (TSB) at ITU. “The application of AI in disaster management must overcome challenges such as algorithmic bias and related data classification disparities, false positives and data generalization errors,” he added.
The new ITU Focus Group on ‘AI for natural disaster management’ will support global efforts to improve our understanding and modelling of natural hazards and disaster risks. The multistakeholder initiative will be supported in close collaboration by ITU, WMO, and UNEP.
“The Focus Group will explore the potential of AI-based algorithms to support data collection handling, modelling of past and future events, such as reconstructions, forecasts and projections; as well as effective communication,” said the Chair of the new Focus Group, Monique Kuglitsch, Innovation Manager at ITU member Fraunhofer Heinrich Hertz Institute.
“High-quality data is the foundation for understanding natural disaster processes, the underlying mechanisms for providing ground truth and calibration data and for building reliable reconstructions, forecasts and projections,” Kuglitsch highlighted. The Focus Group will also consider questions involving the use of AI to generate, monitor and enhance both data quantity and quality. “For instance, how can AI be used to identify objects such as tension cracks on a slope and monitor changes in those objects from remotely sensed images? […] Can AI be used to support the detection of features in real time, such as earthquake signal detection and seismic data? And how can AI be used to leverage synergies from different data sources most efficiently?” asked Kuglitsch. They will also study the criteria for data to be used in training and testing an algorithm. Ethical considerations are also a crucial part to understand the transparency and adaptability of models. Participation in the Focus Group is free and open to all interested parties. Its first meeting is scheduled for 15 to March. Join the mailing list and register as a participant here.
Image credit: Kelly Lacy via Pexels